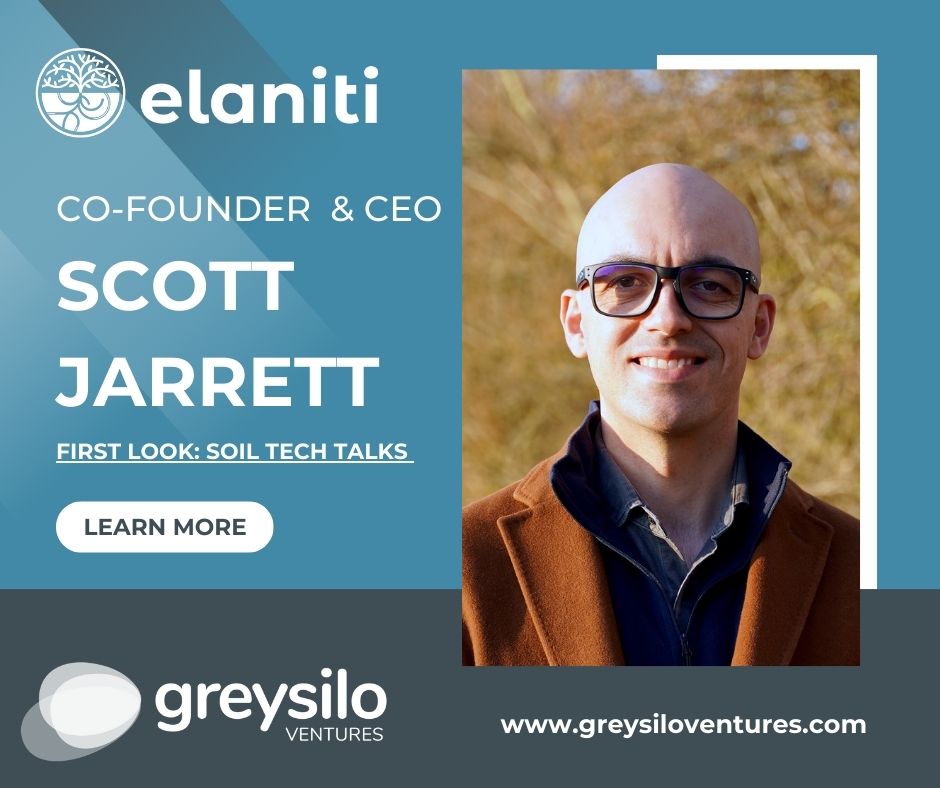
Digging Deeper: How Elaniti’s Soil-Microbiome AI Turns Data into Higher Yields
As we prepare our upcoming Grey Silo Ventures Position Paper on Soil Tech, we’re sharing a series of preview interviews with founders who are redefining how we manage and value the ground beneath our feet. In this first instalment, we speak with Scott Jarrett, CEO of Elaniti, a UK-based start-up that links soil microbiome data to crop performance using machine-learning.
After meeting through the Carbon13 programme dedicated to building ventures for the climate emergency, co-founders Scott and Utkarsh set out to try and address the cause(s) of notable variances in crop performance that had no clear explanation to growers. Based in the United Kingdom, the pair has developed an AI-powered platform linking crop performance outcomes (such as yield and risk of disease) to the soil microbiome. Most recently, the startup announced their partnership with Bayer to use ML techniques to analyze the role of the microbial community in promoting plant growth and resilience.
We know that your solution helps to predict key crop outcomes (such as yield and risk of disease) but how have you quantified/measured the value-add (in terms of ROI for example) of your solution for the farmers?
This is something we are working on with our customers as we speak. Firstly, we had to demonstrate that there was a story to be told to farmers and agronomists with their soil biology data. We did this by proving that our ML models had better predictive accuracy than equivalent models based on soil nutrient data alone. Demonstrating this uplift in predictive performance was the first step, and over the coming years we will quantify that with an ROI by tracking decisions taken (or not taken!) as a result of our forecasts, through to changes in productivity post-harvest.
How does underdstanding the biological composition of soil (soil microbiome) give you an edge in your ability to generate the forecasts and actionable insights for the farmers? Why is the conventional approach (only based on using soil nutrient data alone) insufficient?
Take nitrogen fertiliser as an example. Measuring only soil nutrients (e.g., available nitrogen) overlooks the biological processes controlling nitrogen availability and uptake. Key microbial groups drive nitrogen fixation, nitrification, and denitrification, directly influencing how efficiently plants access nitrogen. By incorporating these microbial dynamics into our forecasts, we provide a more accurate prediction of yield and nutrient use efficiency—something traditional soil nutrient tests alone cannot achieve.
How do the users associate the samples you take with the implementation of the recommendations you give them?
Soil samples that we receive are linked to field conditions, agronomic practices and crop performance outcomes. This allows us to generate targeted recommendations. By tracking implementation and field outcomes, we refine and validate our forecasts over time, ensuring that ongoing soil measurements translate into more precise, actionable insights, ultimately optimising decision-making at the farm level.